If you’ve ever used a Snapchat, Instagram, or TikTok filter, you’ve probably used facial recognition technology. It’s the magic that makes it possible for the filters to put the virtual decorations in the right place, it’s why your beauty filter eyeshadow (usually) doesn’t end up on your cheeks.
It’s fun and cute, but chances are that if you’ve never had any issues with these filters, it’s because your face looks a lot like mine. Unfortunately, the same cannot be said for many other people.
Why don’t zoom backgrounds work for me?
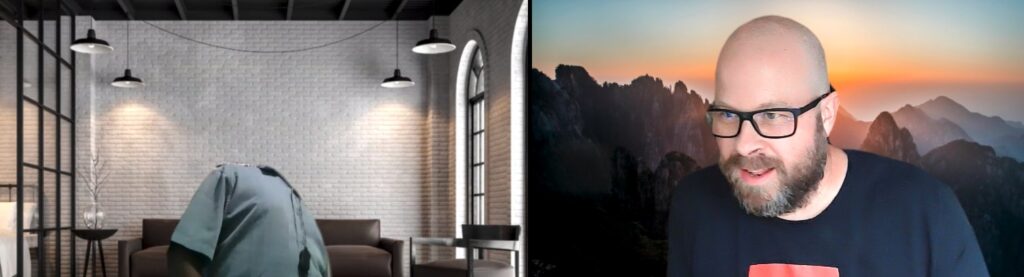
During the pandemic I, along with many other people, used Zoom virtual backgrounds extensively – calm pictures to hide my office background, funny pictures to communicate my current stress levels, you name it. I found they worked fairly well, perhaps struggling a little to handle the edges of my curly hair, but my experience wasn’t universal. When a faculty member asked Colin Madland why the fancy Zoom backgrounds didn’t work for him, it didn’t take too long to debug. Zoom’s facial detection model simply failed to detect his face.
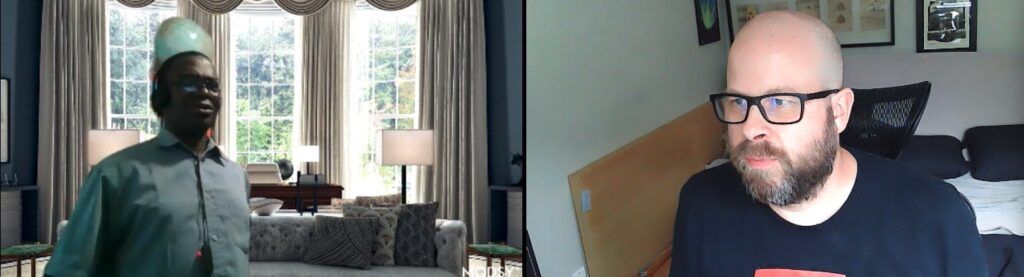
Why can’t I complain about it on twitter?
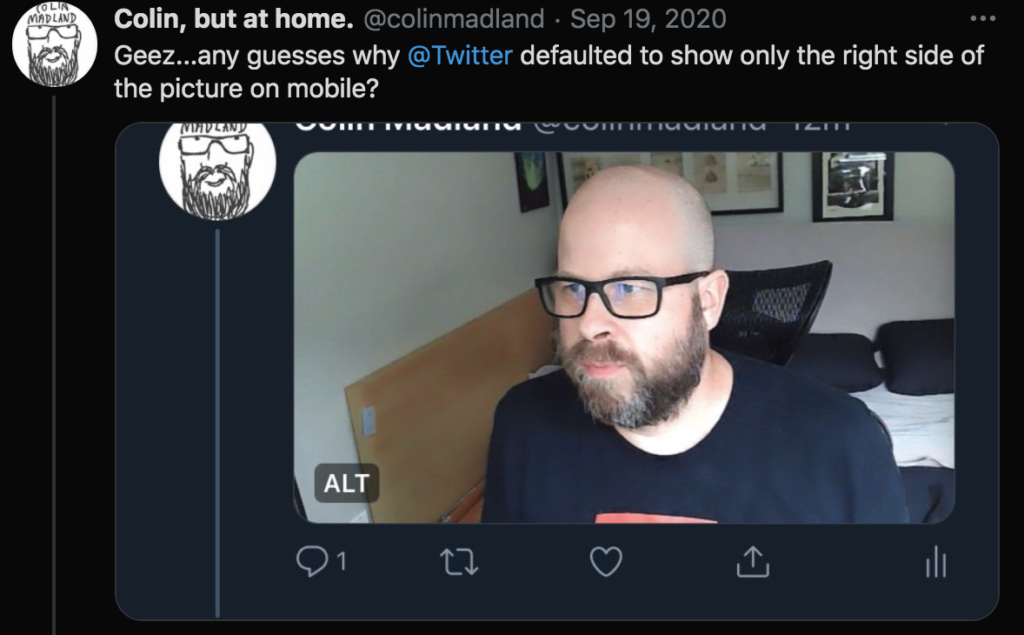
When Colin tweeted about this experience, he noticed something interesting with Twitter’s auto-cropping for mobile. Twitter crops photos when you view them on a phone, because a large image won’t fit on screen. They developed a smart cropping algorithm which attempted to find the “salient” part of an image, so that it would crop to an interesting part that would encourage users to click and expand, instead of cropping to a random corner which may or may not contain the subject.
Guess which part of the image Twitter’s algorithm cropped to. Why did Twitter decide that Colin’s face was more “salient” than his colleague’s?
How does this happen?
Facial Recognition Technology (FRT) is an example of Narrow Artificial Intelligence (Narrow AI). Narrow AI is an AI which is programmed to perform a single task. This is not Data from Star Trek, not the replicants from Blade Runner, this is more like the drinking bird from The Simpsons which works…until it doesn’t.
Facial recognition algorithms are trained to do one thing, and one thing only – recognise faces. And the way you train an algorithm to recognise faces (the way you train any of these narrow algorithms) is by showing it a training dataset – a set of images that you know are faces – and telling it that all the images it sees are faces, so it should learn to recognise features in these images as parts of a face. But there isn’t an “intelligence” deciding what a face looks like, and while it is possible to try and help the algorithm by providing a descriptive dataset, it’s not possible to direct this “learning” specifically. The algorithm is a closed box. Once the algorithm has “learned” what a face is, you can test it by showing it images that do, or do not, contain faces, and see if it correctly tells you that there is a face in the image but, crucially, it is still very very difficult to tell what that algorithm is using to decide if a face is present. Is it looking for a nose? Two eyes? Hair in a certain location?
Is it even looking at the faces at all?
Of course it’s looking at the faces to determine if faces are present, right? How else would it do it?
Well, if you’ve ever done any reading about AI recognition, you’ll undoubtedly be amused by the above statement, because (as I mentioned above) you can’t really specifically direct the “learning” that happens when an algorithm is figuring things out, and in perhaps their most human-like trait, AI algorithms take shortcuts. There’s a well known case of an algorithm trained to determine whether the animal in a photo was a wolf or a husky dog, and by all accounts the algorithm worked very well. Until it didn’t.
The researchers intentionally did not direct the learning away from what the AI picked up the first time it tried to understand the images, and so what the AI actually learned was that wolves appear on light backgrounds and huskies don’t. It wasn’t even looking at the animals themselves – just the photo background. And thus, once it was tried on a larger dataset, it began incorrectly identifying wolves and dogs based on the background alone.
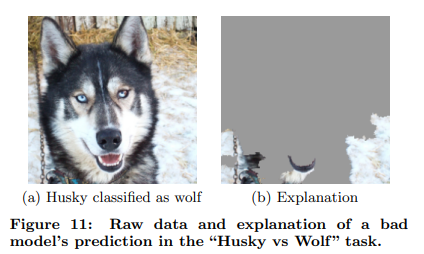
This situation might have been constructed just for research, but the hundreds of AI tools developed to help with Covid detection during the pandemic were not, and as you may have already guessed, those algorithms by and large were not the solution to the problem, and instead had the same problem as above.
A review of thousands of studies of these tools identified just 62 models that were of a quality to be studied, and of those, none were found to be suitable for clinical use due to methodological flaws and/or underlying bias. Because of the datasets used for training these models, they didn’t learn to identify Covid. One dataset commonly used contained paediatric patients, all between age 1-5. The resulting model learned not to identify Covid, but to identify children.
Other models learned to “predict” Covid based on a person’s position while being x-rayed, because patients scanned lying down were more likely to be seriously ill and the models simply learned to tell which x-rays were taken standing up and which were taken lying down. In some other cases the models learned what fonts each hospital used and fonts from hospitals with more serious caseloads became predictors of Covid.
And there are so many other incidents of AI failing to recognise something correctly or failing to have some human oversight or context applied that the AIAAAC has an entire repository to catalogue them.
What has this got to do with Facial Recognition Technology in Ireland?
Quite a lot. I’ve already shown you that AI models are often not very good at recognising the things they are supposed to be trained to recognise. Much of this is down to the training dataset that you use – the pictures you show your algorithm to teach it how to recognise things.
You may have already spotted a problem here because, of course, not all faces are alike – some people have facial differences that may mean their face does not have a typical structure, some may have been injured in a way that has changed their face. And, of course, the world contains a wide variety of skin tones. And if you are asking if the kinds of datasets available to train these models contain a diverse set of faces, incorporating all of the above and more, then the answer is a resounding no. Efforts are being made by some groups to address this, but progress is slow.
And what this boils down to is this: if your training dataset only contains typical faces, then your facial recognition algorithm will only learn to recognise and identify typical faces. If you train your facial detection models using data that isn’t diverse, you will release software that doesn’t work for lots of faces. This is a well known, pre-existing, and long running problem in tech.
Moreover, if you are not careful with your dataset, you will reinforce existing stereotypes and bias, a problem which is more severe for certain groups, such as women and POC. Models don’t just learn what you want them to learn, they also learn stereotypes like “men don’t like shopping” when they are trained on datasets in which most shoppers are female.
Can’t we put in some checks and balances, some safeguards?
Well, yes and no. While people are working to help people improve datasets, there are many datasets out there which contain demographic information which will likely be used to help train and build national or large-scale models, and since this demographic information represents how things currently are, it will also represent how people have been impacted by existing or past biases (e.g. historically poorer parts of a country, or groups of people who have been oppressed and, as a result, have lower rates of certain demographic indicators such as college education or home-owning). It’s hard to escape these biases when they are built into the data because they are still built into our societies.
Additionally, in order for there to be checks and balances, we would need those in charge to understand the implications of all of the above (and more) and to care enough to enforce them by writing legislation with nuance and skill. This is a complex area that has caused issues in many countries that have tried to adopt some form of FRT.
We have examples in our own country’s recent history about how our government has legislated for (and cared about) personal and biometric data, and their record is not good. An investigation by the Data Protection Commission found significant and concerning issues with the way the Public Services Card had been implemented, and the scope expanded without oversight or additional safeguards, sharing data between organisations that were never intended to be in the scope of the card. The Commission said, in its findings, that “As new uses of the card have been identified and rolled-up from time to time, it is striking that little or no attempt has been made to revisit the card’s rationale or the legal framework on which it sits, or to consider whether adjustments may be required to safeguards built into the scheme to accommodate new data uses.” The government’s first response to this report was not to adjust course or review the card internally itself, but to appeal this ruling and continue to push the card without any revisiting. (They dropped the appeal quietly in December 2021).
On this basis, I do not believe that the government in its current form has the capacity or the will to legislate safely for the use of FRT. A first foray into gathering public data en masse resulted in illegal scope creep, extending the card’s reach far beyond what was permitted without any announcement or oversight, and no review or change to the safeguards. This is something that simply cannot be permitted to happen when it comes to the use of facial recognition technology, which has the potential to be infinitely more invasive, undermining rights to privacy and data protection, and (with flawed datasets) potentially leading to profiling of individuals, groups, and areas.
Facial recognition technology is not fit for purpose. Existing models are not good at recognising a diversity of faces, and are unable to account for the biases built into the datasets that are necessary to train and build them. It cannot be a one-stop solution for enforcing laws.